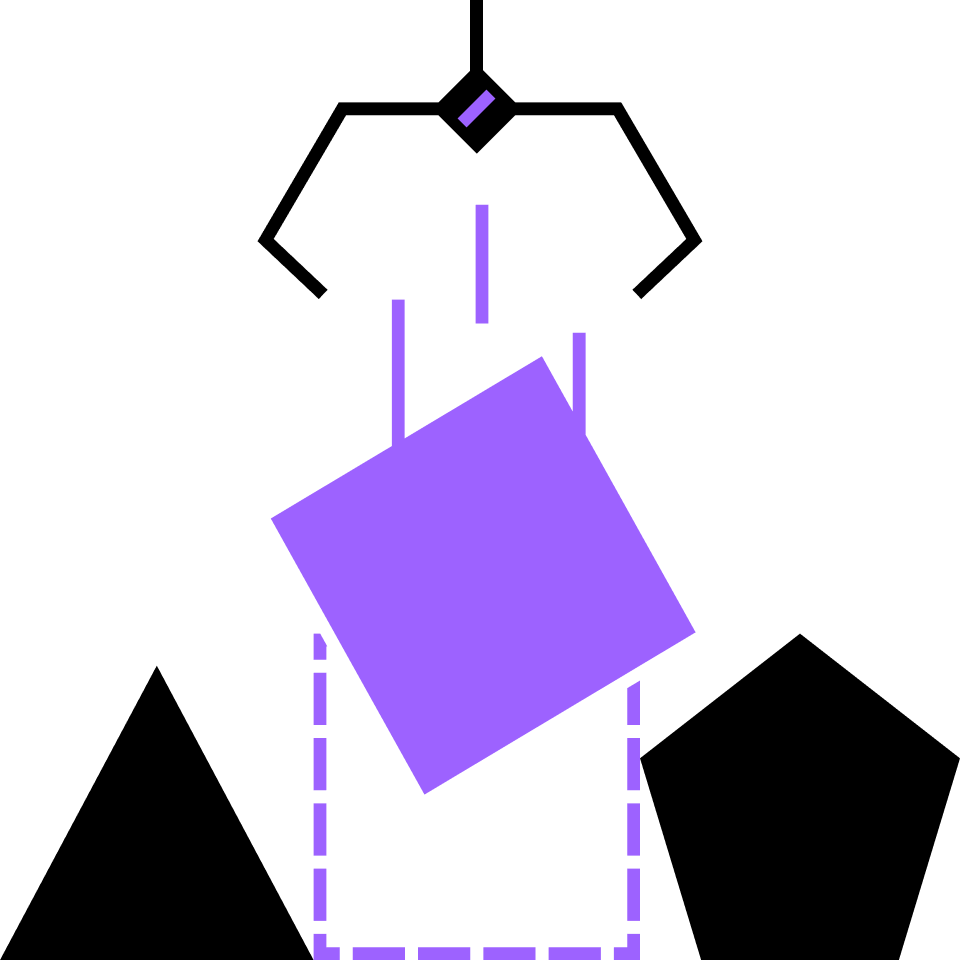
CS & Programming · Level 3
3.2 Introduction to Algorithms
Learn how to make a computer do what you want, elegantly and efficiently.
Pseudocode
Conditional Algorithms
Repetition
Manipulating Numbers
Arrays
Searching an Array
Binary Search
Sorting an Array
Insertion Sort
The Stable Matching Problem
Using Greediness
Deferred Acceptance Algorithm
Correctness
Termination
Variants
Course description
An algorithm is a step-by-step process to achieve some outcome. When algorithms involve a large amount of input data, complex manipulation, or both, we need to construct clever algorithms that a computer can work through quickly. By the end of this course, you’ll have mastered the fundamental problems in algorithms.
Topics covered
- Pseudocode
- Variables
- Conditionals
- Repetition
- While loops
- For loops
- Binary search
- Selection sort
- Insertion sort
- Stable matching
- Algorithmic complexity
Prerequisites
Up next
4.1 Algorithms and Data Structures
The fundamental toolkit for the aspiring computer scientist or programmer.
Jump ahead